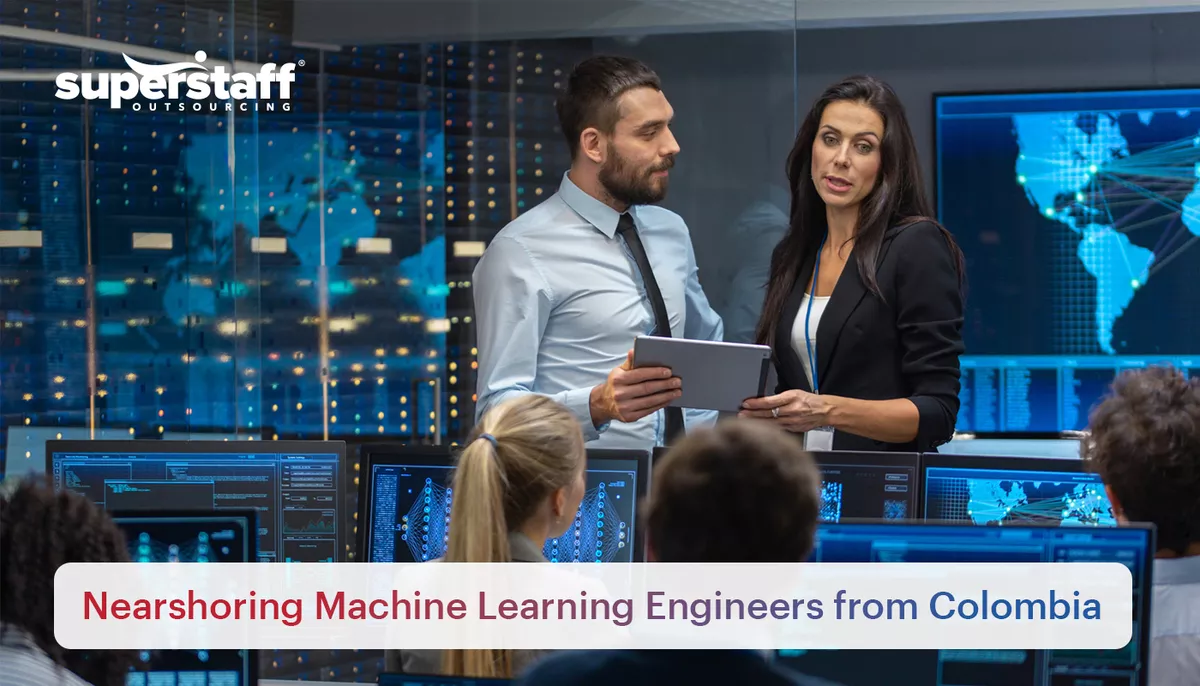
2023 marks a pivotal moment for generative AI, with machine learning (ML) emerging as one of the most coveted innovations in today’s industry. Tech giants are now harnessing the technology’s capabilities to enhance their business operations, strengthen customer relations, and automate critical processes after recognizing its massive potential in the current market.
As more organizations integrate ML into their workflows, what can businesses do to gain momentum and accelerate growth? Nearshore machine learning engineer teams from Colombia.
5 Compelling Statistics Showing Demand Spikes for Machine Learning
More and more companies are hopping on the AI hype, driving higher demand for machine learning engineer jobs to navigate the increasing complexities of ML models — and we have the stats to prove this:
- AI and ML experts are the most sought-after roles in 2023.
- The demand for AI and ML specialists increases by 40% as the adoption of high-performance tech becomes widespread.
- In the U.S., a machine learning professional earns an average salary of $151,233 annually, about $90,000 higher than the standard wage nationwide.
- By 2030, the global ML market value will hit $225.91 billion from $26.03 billion in 2023.
Even industry analysts and tech executives are trumpeting AI tools as the next big thing, comparing it with the advent of the internet and electricity. So, in an era of rapid technological advancements and crippling labor shortages, businesses must invest in data-driven solutions and hire ML engineers from Colombia.
Why Is There a Growing Demand for Machine Learning Engineers?
Growing Complexity of Machine Learning
You see examples of machine learning everywhere without even realizing it.
Using statistical models and algorithms, ML enables computers and software to study patterns and relationships and create predictions and decisions. Some ML examples are the music, videos, or movie recommendations you receive from Spotify, YouTube, and Netflix. ML algorithms associate user preferences (such as videos, saved songs, or subscribed artists) with another with similar interests or tastes.
However, ML requires constant fine-tuning of hardware and capability enrichment — skills mostly found in data scientists — to enhance accuracy and rectify errors. Given that more companies are working with advanced tech solutions, the Bureau of Labor Statistics predicts that the demand for data scientists will spike faster than most professions, with a projected 23% growth rate by 2032.
Apart from needing data scientists to input and train data, firms also need machine learning engineers — a support squad for the data science department.
Applying the “deep learning” model, these engineers layer the structure of algorithms and deploy mathematical operations, determining user patterns more precisely. In short, they make software process data like a human brain would.
Accessibility of Machine Learning
The advent of user-friendly, no-code, and low-code platforms has democratized machine learning, making it more accessible even to non-experts. Nowadays, people with minimal coding experience can create and deploy models, opening up opportunities for innovation across industries.
This, in turn, spurs a virtuous cycle. As more non-experts adopt ML, it will give rise to the demand for more user-friendly systems and applications — and developing these calls for machine learning engineering skills from specialists.
One such innovation includes the central cloud, which offers accessible machine learning services that provide pre-built models and easy-to-use APIs. Consequently, businesses can eliminate the need for extensive infrastructure and leverage the power of ML without significant upfront investment.
Moreover, open-source libraries like TensorFlow and PyTorch have made high-quality machine-learning tools available to anyone with an internet connection, enabling developers to build sophisticated models and solutions without starting from scratch.
The Great Resignation
The tech industry is notoriously understaffed, making it a growing problem in the U.S. and worldwide.
The quit rate among IT and tech professionals is also spiraling out of control, with:
- 10.2% lower intent to remain in their jobs than non-IT workers — the lowest of all business roles.
- 72% have considered resigning over the past 12 months.
In contrast, many businesses are working towards minimizing time-consuming and monotonous work, with two-thirds of firms at least testing the automation processes in one or more units or functional areas — compared to 57% in 2018. The same report also found that 65% of respondents from smaller businesses are successful with their automation efforts.
How will businesses be able to bridge this discrepancy?
Businesses can outsource machine learning engineers and data scientists to mitigate the effects of labor shortage. With their advanced skills in statistics and computer science, they can quickly develop ML-automating tools to speed up business operations, streamline workflow, and improve work productivity.
Consequently, ML engineers will continue to gain appeal as more businesses turn to automation.
What Does a Machine Learning Engineer Do?
The complete reliance on your “gut” feelings won’t cut when making business decisions. Indeed, an intuitive mind can be a sacred gift. Still, it’s through data that businesses unlock meaningful insights rooted in quality, accuracy, and credibility — and ML engineering can be the key to this.
Then again, the machine learning engineer vs. data scientist comparison is a tall order. While data scientists focus on organizing, processing, and deciphering big data sets, ML engineers leverage algorithms when evaluating large amounts of data, learn from it, and predict upcoming trends.
Here are some of the critical roles of ML engineers:
Implementing ML Algorithms
Businesses strive to drive down costs, especially when economic uncertainty remains elevated. All the while, they don’t want to jeopardize their work quality.
Entrusting the adoption of ML algorithms to these engineers enables your existing software and infrastructure to utilize historical data, make accurate predictions, and automate some manual labor — and ensure its seamless integration. Several industries have reaped the benefits of this innovation, such as:
- Healthcare: Diseases and risks identification in the healthcare sector
- Banking sector: Fraud and scam detection
- Travel and hospitality: Price and cost estimation
- Automotive industry: Innovation of self-driving cars
- Social media: Relevant recommendations on feeds based on keywords
One common type of ML algorithm is the linear regression model, a subset of predictive analysis. This predictive modeling estimates the value of a dependent variable based on an independent variable. In business, the model can estimate future sales (dependent variable) based on the sales performance for the past few months (independent variable).
Testing AI Systems
A few years ago, an Arizonan woman died in a fatal collision with a self-driving Uber because of its inability to recognize the pedestrian. In a recent trial, the driver pleaded guilty and was sentenced to three years’ probation — the first-ever deadly self-driving car crash in history.
This is just one example of the consequences of AI systems without proper ML engineers’ testing.
Adhering to rigid standards and procedures, these professionals assess the practicality, accuracy, and resilience of your software and infrastructure, ensuring their continuous and safe usage in the long run.
Other tests they take to improve AI-powered solutions include:
- Backtesting: Comparing various past strategies. Techniques that did poorly in the past are unlikely to perform well in the future
- Turing test: Determining whether software can demonstrate human intelligence
- Systems integration testing (SIT): Ensuring that the AI system can function well with other networks
Designing and Developing Machine Learning Systems
By 2023, a McKinsey study projects that efficient workflows and seamless communication between humans and software will be the standard as businesses become more data-driven, implementing ML systems imperative. However, designing a reliable, adaptive, scalable ML architecture isn’t easy. It requires extensive data analytics and mathematics knowledge — a niche in ML engineers.
In doing so, these professionals will contextualize machine learning in your company and select appropriate algorithms based on its specifications and core functions. Then, they will customize, design, and optimize ML models to suit your unique business needs.
Performing Statistical Analyses
As mentioned, the data-driven culture has taken center stage in many corporations. With abundant data, business executives must use them to their advantage and accelerate growth.
As such, ML engineers can execute statistical analyses to assess and train immense data and then interpret them to forecast future market trends. Businesses can develop suitable marketing and sales strategies to attract, as well as retain, prospective customers by using the gathered intelligence.
Considering that excessive data exposure is the third most critical application programming interface (API) threat in 2019, the engineers can also filter out and eliminate certain information incongruous with public exposure — creating a more harmonious data organization.
How To Find a Reputable Nearshore Partner
The “silent revolution” of ML is fueling the demand for professionals apt in machine learning skills. Yet, ML prowess is hard to find — and ML engineers are in short supply.
Instead of heavily relying on sourcing and hiring for the right talent amid labor scarcity, businesses can collaborate with a nearshore partner renowned in the tech field.
However, while nearshoring can be a great “fix,” it’s still a big decision capable of propelling your business forward or causing permanent damage. Hence, companies must observe best practices when selecting a nearshore partner to deliver the best possible outcomes.
Research and Due Diligence
When exploring potential partners, you must first understand your business needs. Evaluate your overall business operations and search for areas that can be improved further. Then, ask yourself if implementing ML can further enhance your business functions. If so, you can go ahead with your research.
Here’s a to-do list for you when researching for an ideal nearshoring partner:
- Study the landscape of outsourcing providers, focusing on their reputation, expertise, and client feedback.
- Verify if the outsourcing company has a track record in machine learning engineering and possesses the necessary technical skills.
- Seek reviews from previous clients to gauge the partner’s performance and reliability.
- Ensure the outsourcing partner complies with relevant data protection and privacy regulations.
- Assess if the partner can scale their team and adapt to your project’s changing needs.
After these steps, don’t just stick with one potential vendor. Pro tip: always ensure you have at least four to five top candidates in your lineup.
Conducting Interviews and Assessments
An effective interview is the core of choosing the right nearshoring partner. While you’ve read all reviews and testimonies, there are possibilities that they may be self-serving or, worse, self-curated to hide their incompetence. So, talking with them and assessing their capabilities upfront is always best.
Here are some guidelines for you when interviewing your candidates:
- Engage in technical discussions to evaluate their proficiency in machine learning concepts, algorithms, and frameworks.
- Assess if their work culture aligns with your company’s values and work ethic for seamless collaboration.
- Effective communication is crucial. Ensure there are no language barriers that might hinder project progress.
- Pose real-world scenarios or challenges to gauge problem-solving skills and creativity.
Why Nearshore Machine Learning Engineers From Colombia?
Recognizing the sizable contribution of the BPO industry to Colombia’s economy, its government has enacted several favorable regulations to attract U.S.-based investors. Owing to these initiatives, this Latin American nation has become a top destination for nearshoring, especially for tech-related functions.
If you’re looking to outsource ML engineers for your firm, Colombia is always your best bet — and here’s why:
Tax Benefits for Tech-Related Industries
Research, technological development, or innovation investments enjoy a 30% tax credit to resolve commercial challenges, encourage scientific advancements, and explore new markets. As such, U.S. investors can drive down liabilities and fund initiatives to future-proof their business operations.
Qualified Labor Pool
Colombia’s national educational innovation ecosystem is fueling new waves of young, talented, motivated individuals and tech-related experts.
For instance, the government introduced Aprendizajes para el futuro, designed to foster reliable digital landscapes, fine-tune innovative skills, and encourage scientific development among the youth. Many Colombian students have come to possess strong IT and software development expertise, preparing them for the rising need for ML engineers.
Thriving Location for Tech Services
Medellin has now established itself as one of the world’s most innovative, safe, and tourist-friendly cities. Recently, the city embarked on numerous smart-city projects to improve the livelihood of Colombians and compete with global markets.
One such program is the “Medellín’s El Cinturon Verde Metropolitano” or Metropolitan Greenbelt, aiming to address suburban slumps and food insecurity. The plan proposes building recreational spaces, hiking trails, and systematic housing, which is expected to be completed by 2030.
Harness the Power of AI With a Nearshore Machine Learning Engineer Team
In today’s data-driven world, unlocking the potential of Artificial Intelligence (AI) is crucial for staying ahead in the competitive landscape. Our nearshore Machine Learning Engineer team combines expertise with cost-effectiveness, ensuring you have the right talent to drive your AI initiatives.
Contact us today and embark on a journey of innovation and efficiency with our dedicated team of experts. Don’t miss the opportunity to revolutionize your business — seize it now!