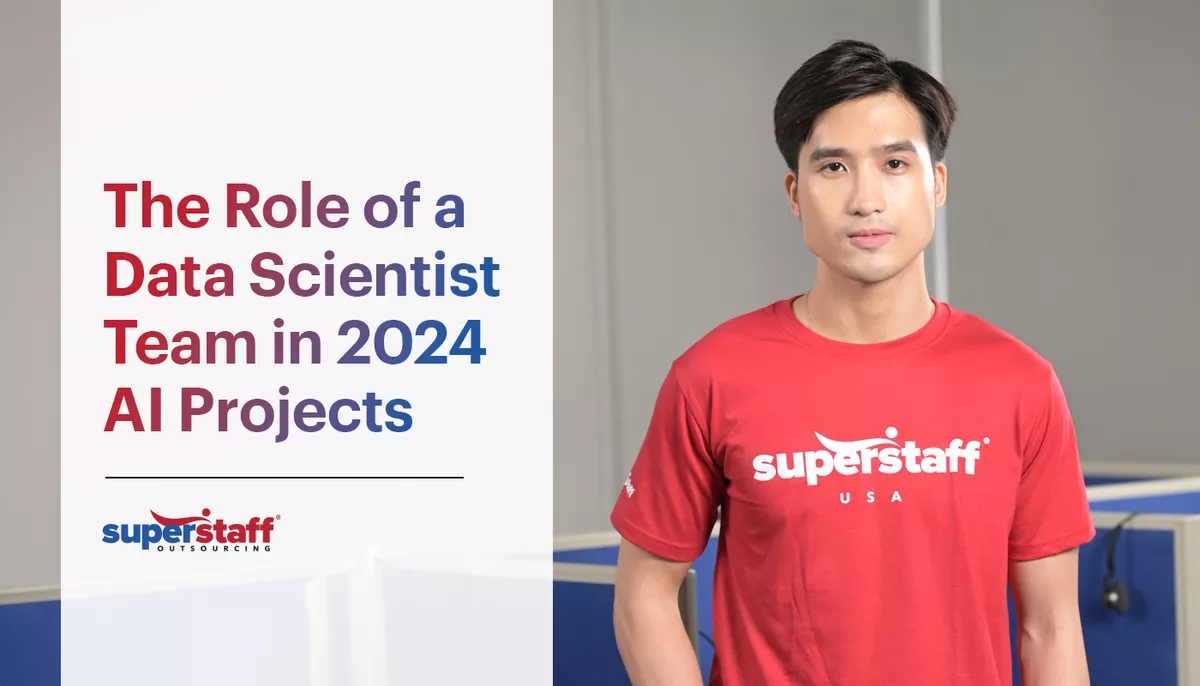
During the edgy 90s, an era famous for the dot-com boom, computer engineers and Wall Street “quants” were the hot roles in the business.Â
Today, in the Age of Artificial Intelligence, data scientists have been hailed as “the sexiest job of the 21st century.”
AI and machine learning solutions have become increasingly important across various industries, setting the stage for the thriving growth of data science.Â
So, what is a data scientist, and how can they empower AI development? Let’s find out.
The Role of Data Scientists on AI Development
At the core of AI development lies data — the lifeblood fueling algorithms and models. However, data in its raw form is an uncut gem: full of potential but still needing refinement. This is where data scientists step in, meticulously processing and cleaning data into a form suitable for analysis. Their expertise involves understanding the data’s structure and extracting insights from raw information.
To delve deeper into our topic, let us answer this question: What do data scientists do to help design a robust AI structure?
Navigating the Complex Terrain of Algorithms
Before data scientists extract insights from unorganized, chaotic data, they must formulate a series of commands–or algorithms–directing the computer to execute precise actions, streamlining complex procedures.Â
Algorithms are the backbone of AI systems, enabling machines to learn, predict, and make decisions. Data scientists are adept at navigating this complex AI terrain, employing many algorithms, from classical machine learning techniques to cutting-edge deep learning models. They select the most suitable algorithms for a specific project to achieve optimal performance.
Training AI Models
Training AI models is an ongoing process that necessitates constant evolution and adaptation. As new data sources emerge and existing ones evolve, the AI system’s appetite for diverse data grows, securing its spot at the cutting edge of innovation and relevance.
Say you own an online retail store. Data scientists can collect customer data and use them to create accurately tailored recommendations for each client based on their buying history and browsing habits. To take it a step further, they will conduct surveys to determine whether the clients are satisfied, advancing more data into their AI models.
Harnessing Data Feedback
Evaluating your AI system’s performance isn’t a mere formality; it’s the litmus test of your AI capabilities across a spectrum of tasks. Before a company launches the system, data scientists will put it through several rigorous challenges and scenarios that mirror real-world applications. They employ various metrics to assess the model performance, including precision, accuracy, and recall, to discover its strengths and highlight areas that demand refinement.
For example, a data science professional will test whether a self-driving car’s AI system recognizes the road’s every turn and identifies different road obstacles (a stray shopping cart or a person in a wheelchair). If it passes the test, the subsequent testing stages follow until the model reaches perfection.
Data-Driven Deployment of AI Systems
The transition from lab to production represents the deployment of your AI/ML system. However, the journey doesn’t end at deployment; continual vigilance and enhancement are critical for effective AI development.Â
Monitoring the system post-deployment is paramount to ensuring its continued efficiency and reliability. Here, data assumes the role of a vigilant overseer, constantly observing the system’s behavior and performance over time.
Notably, data-driven monitoring isn’t solely about passive observation; it’s an active process that involves analyzing incoming data streams to gauge the system’s health. Data science professionals monitor anomalies, deviations, and performance metrics to stay ahead of potential issues. This proactive approach allows swift interventions and refinements, ensuring the system stays aligned with its intended functionality.
Strategies for Success: Best Practices for Data Scientists in AI Development
Undeniably, data science will become the next big revolution in 2024. More and more industries are witnessing its potential in the business world, with almost 60% of organizations employing 50 or more data scientists between 2020 and 2021.
But considering the intricacies behind data science jobs, a data engineer or scientist must possess an in-depth understanding of data processing, including engineering, extracting, manipulating, and analyzing data. Otherwise, inconsistencies and inadequacies in projects will be inherent.
Hence, companies must adopt best practices to produce and improve data science projects. Let’s explore these strategies for data scientists to build state-of-the-art AI models.
Define the problem clearly.
Before delving into the intricacies of data and algorithms, it’s crucial to grasp the problem you aim to solve or the question you seek to answer. Defining the problem and outlining your goals for AI development significantly minimizes confusion, ambiguity, and unnecessary efforts.
Here are some guide questions you can ask yourself to craft a perfect problem statement:
- What problem am I trying to solve?
- What is my goal, and does it align with my problem?
- Who are my stakeholders and audiences?
- What should I do to attain success and eliminate constraints?
Establishing these questions can set the stage for your analysis, helping you channel your energy into solving the problem you need to address.Â
Another pro tip is to immerse yourself in the domain related to your problem. This may be daunting, but it helps contextualize your situation — a crucial step in framing the issue and selecting proper analytical methodologies for resolution.
Build a dedicated program for data science in the organization.
Here’s a stark reality for businesses: most are bound to fail if they do not understand their market’s needs. As such, monitoring customer behaviors through data science can help you collect vital insights into your target market’s desires and preferences.
 To do this, establishing a cross-functional data science team comprising diverse skill sets — including data engineers, machine learning experts, and data analysts — with documented operational procedures is essential. This approach enables companies to realize their full potential and unearth concealed opportunities.
Invest heavily in data models.
The adage goes: “The best time to repair a roof is when the sun is shining.” In other words, the best time to invest in your data models is while you’re successfully integrating these systems into your daily operations. Your data models should evolve with the dynamic business objectives and goals.Â
The way to do this is by establishing transparent and consistent data policies and standards, including complying with local and federal legislation. Ensure your AI projects are aligned with your business goals and consumers’ needs by setting relevant KPIs and metrics. With quality data in your systems, you’ll achieve a 360-degree view of your demographics, enabling you to deliver seamless transactions and offer tangible value to your customers.
Collaborate with other teams and stakeholders.
Building an AI model from scratch can be impractical, especially when you lack domain expertise. Mastering these skills takes years of experience and intensive training, with some schools offering tertiary education for data science.
The optimal solution lies in close collaboration with different teams. Working with data engineers and business stakeholders ensures seamless data flow and alignment of objectives. This tight-knit teamwork enables multiple perspectives to converge on actionable strategies that enhance AI systems while identifying critical metrics for sustained success.
Communicate openly and transparently.
A report ranks communication (24%) as one of the most durable soft skills in 2023. While technical skills help you complete the job, your soft skills are the ones that fuel customer attraction and retention. Warren Buffett, an acclaimed billionaire and investor, expressed that honing your communication skills can level up your worth up to 50%.
So, remember: Not all of your stakeholders are data science experts. When communicating your AI development ideas to these stakeholders, leverage data visualization and storytelling techniques to convey complex insights and ensure clarity and conciseness. If you suspect any confusion or misunderstandings, address the issue head-on and ask the right questions.
Incorporate an agile methodology.Â
An agile methodology hinges on these three tenets: timely delivery, adaptability to unforeseen changes, and automation.
In other words, data analysis and model development processes must focus on continuous improvement and adaptation to changing requirements.Â
Allowing customers to view the project’s progress will also help data scientists provide a non-technical overview. This, in turn, fosters trust and accountability as businesses can modify and alter some parts of the project upon the client’s requests.Â
Leverage standardized tools.
Excelling in the data science field necessitates using industry-standard tools to ensure all team members’ compatibility and ease of use. These standardized tools offer a consistent data analysis and modeling environment across teams and projects, facilitating reproducibility and streamlining work collaboration.
What are the essential aids you should keep in your data science toolbox? Here are three of them:
- Programming languages: Write code for easier data manipulation, analysis, and machine learning. Despite “Python” and “R” being the more popular languages, expanding your range can enhance your system’s versatility.
- Data wrangling: Clean, transform, and prepare massive amounts of raw data for analysis and extraction.
- Version Control: Detect changes, create avenues for model experimentation, and consolidate different versions of your code.
Ensure data privacy and security.
In 2023, the average data breach costs hit $4.45 million worldwide, a 2.3% spike from 2022. But it’s not all bad news. Over half (51%) of organizations are augmenting their security investments to minimize cyber threats and defend large amounts of data.Â
The importance of data privacy and security can’t be understated, especially with regulations across the U.S. mandating companies to safeguard critical and confidential information.Â
Fortunately, data scientists can implement robust security measures to protect sensitive data, maintain privacy while complying with state and local privacy legislation, and ensure ethical data science practices. These specialists can anonymize and encrypt vulnerable information and enforce access controls to safeguard databases from unauthorized cyber entries.Â
Furthermore, they can install AI security, which emulates human prowess in detecting fraudulent activities. According to the same study cited above, companies implementing AI security reported $1.76 million lower data breach expenses than those that did not adopt such measures.
Continuously learn and adapt.
The ever-evolving nature of the data science field is inevitable, making continuous learning and adaptability a must for data professionals. They must constantly update their niche knowledge in AI and ML advancements and keep pace with the latest methodologies, tools, and techniques. Doing so requires regularly attending specialized conferences, workshops, and webinars and engaging in online dialogues with data science communities.
Simultaneously, data science specialists must cultivate a culture of experimentation and innovation to explore new techniques and approaches.Â
Let’s say your goal is to diversify your audience reach and target a specific demographic that doesn’t necessarily purchase from you. Will they be interested in my products? Will this strategy expand my market? Is this risk worth taking? You can only validate your answers once you put these hypotheses into practice. Â
Outsource data scientists.
The Bureau of Labor Statistics predicted a 35% rise in data science employment from 2022 to 2023, higher than the average for all sectors. Additionally, nearly 17,700 data scientist jobs are open for recruitment, as many are already departing the workforce.
With artificial intelligence and ML dominating the business realm, companies must adopt strategies that support uninterrupted staff supply. Here, outsourcing is the key that unlocks access to a diverse pool of specialized talent.
Data science outsourcing firms often have teams of experts in machine learning, AI, statistics, and other domain-specific knowledge, providing a wide range of skills that may not be available in-house. With their expertise and experience, outsourced data science teams can leverage their skills and resources to expedite development and implementation for quick data-driven solutions.
Outsourcing data science can also be cost-effective. Instead of investing in hiring and training an entire in-house team, outsourcing offers flexibility in scaling resources as needed, reducing operational costs associated with staffing and infrastructure.
Harness Outsourcing’s Potential: Key Advantages of Nearshore Data Scientists from Colombia
In the dynamic landscape of data science, sourcing talent has become a strategic endeavor crucial for leveraging data’s transformative power. Amidst this pursuit, the emergence of nearshore talent has garnered attention, with Colombia shining as a hub for exceptional data science expertise among the burgeoning options.Â
The allure of nearshore data scientists from Colombia extends far beyond geographical proximity, offering many advantages that propel businesses toward innovation and success, such as:
Rich Staffing Pool and Technical Expertise
Colombia’s growing tech experts have quickly satisfied the soaring demand for tech and IT, positioning the nation at the forefront of data scientist nearshoring.Â
With 53 Colombian educational institutions featured in LatAm’s top 400 LatAm universities, the country continues to produce a high-quality workforce with diverse skill sets ranging from machine learning and predictive models to big data technologies.Â
Moreover, the ICT Mission 2022, a program launched by President Iván Duque and ICT Minister Karen Abudinen, trains 100,000 Colombians with competitive skills related to software development and programming.
Cultural Affinity and Collaboration
Colombia and the U.S. have been close allies for decades, sharing diplomatic relations and collaborating to overcome challenges, including prosecuting transnational criminal activities, alleviating immigration perils, and creating more employment opportunities.
These shared goals nurtured their deep connection and helped integrate both countries’ multinational companies seamlessly, fostering a cohesive and smooth work environment.
Cost Efficiency and Competitive Pricing
As staff shortages reverberate across the tech sectors, business owners must seek ways to address this challenge while innovating operations and optimizing costs. Thankfully, Colombia nearshoring offers a favorable cost structure compared to onshore options, providing cost-effective solutions without compromising quality.Â
To illustrate, U.S. data scientists earn an average of $8,801 monthly. Colombian data science experts receive $3,257 (COP 13M). Furthermore, the nearshoring model doesn’t incur additional charges apart from the agreed-upon monthly/hourly rate on the service-level agreement (SLA).Â
Language Proficiency and Communication
Colombia’s EF English Proficiency Index (EF EPI) has improved significantly since 2020, placing it at a competitive advantage with English-speaking clients. Equipped with outstanding English proficiency, Colombian data scientists can convey their thoughts accurately in technical discussions, further facilitating efficient collaboration and mitigating language barriers.
Time Zone Synchronization and Agile Workflow
Colombia’s geographical positioning offers more than just a strategic trade advantage. Being in the Eastern Time Zone enables real-time interaction and reduces communication delays between U.S. and Colombian teams. Without substantial time differences, both teams can sustain prompt and responsive feedback cycles, guaranteeing that all project deadlines will be met.
Innovation and Adaptability
Boasting over 380 top-tier software development companies, Colombia has remained a pivotal tech hub in the LatAm region. Coupled with its exceptional labor productivity growth and sophisticated training, Colombian data scientists have acquired the ability to swiftly adapt to new technologies, methodologies, and business landscapes, positioning themselves as versatile assets in navigating the evolving data science landscape.Â
Government Support and Infrastructure
The growing confidence in Colombia’s government support is evident, with the country scoring 85% for the willingness to participate in open forums and 80% in enacting legislation conducive to BPO companies. One notable government initiative involves improving internet infrastructure, enabling nearly 70% of the local population to enjoy internet access. This strong support bolsters the growth of data analyst and science capabilities, ensuring a robust ecosystem for talent development and industry advancement.
Experience the Transformative Power of Colombian Data Science Expertise
The decision to hire a data scientist team from Colombia transcends geographical proximity; it’s a strategic investment in accessing top-tier talent aligned with global industry standards. The blend of technical prowess, cultural affinity, cost efficiency, and adaptability positions Colombia as a compelling BPO destination for businesses seeking to harness the power of data science.Â
Embracing this nearshore advantage unlocks the potential for innovation, efficiency, and sustained growth in today’s data-driven landscape.Â
Partner with SuperStaff now, and allow us to transform your AI operations. Our strong BPO presence in Colombia ensures exceptional service while allowing your business to focus on growth.Â