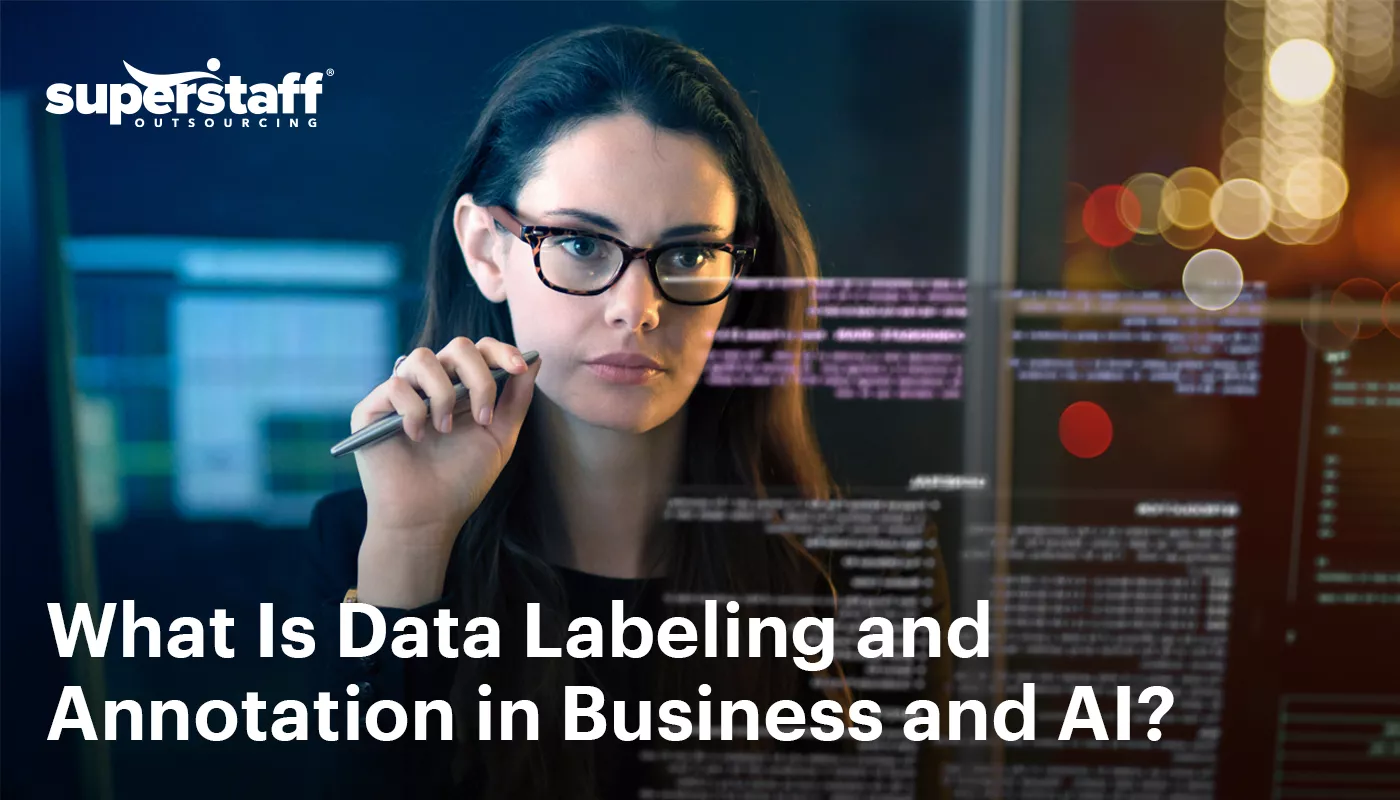
The explosion of Artificial Intelligence (AI) and Machine Learning (ML) models stems from one fundamental component: data. Many still undermine its significance in propelling tech advancements to greater heights.
Data is indeed the critical cog that empowers innovations — and at the heart of this lies data labeling and annotation. These processes are the key to organizing and tagging raw data, guaranteeing its high quality and accuracy for optimal desired results.
So, what is data labeling and annotation, and what are its best practices to further enhance AI and ML systems? Let’s delve into some of the insights.
The Crucial Role of Data in Business Strategies
Data reigns supreme in today’s digital era, serving as the cornerstone for business operations across several industries. It isn’t just about numbers; it’s more about the story it tells, the insights it reveals, and the opportunities it unlocks. Businesses strategically harnessing data’s power will continue to innovate, evolve, and thrive in an increasingly competitive landscape.
Just take a look at these compelling numbers:
- AI adoption is gradually thriving, with 35% of companies already using AI and 42% exploring its benefits
- 56.5% of companies fueled innovation with data
- 91% are investing in AI initiatives
- Most respondents (54%) agree that AI automation leads to cost savings and efficiencies
- The global market size of AI is expected to hit $2,575.16 billion by 2032
What Is Data Labeling and Annotation
Structured data isn’t just a “nice to have” anymore. With millions of raw data points levitating across your database, businesses need skilled human annotators to annotate, categorize, and identify them with informative labels.
So, what is data annotation and labeling?
Consider this scenario: how do you ensure things are in their designated spots at home? You label them. Doing so establishes a clear understanding of where each item belongs. This organization saves valuable time, allowing you to instantly and systematically find items without extensive searches.
Now, let’s apply the concept to your smartphones. You just came from several relaxing trips and took tons of photos. You will need to tag all the images with keywords such as “beach,” “mountains,” or “friends,” a form of data labeling that allows you to find them in the future quickly. This way, you won’t waste time browsing your gallery to search for your favorite picture.
Besides image annotation, you can also label items in videos with relevant identifiers. This is especially helpful for AI-powered self-driving cars. Enormous amounts of video data train the self-driving vehicle to navigate traffic and avoid road obstacles. For its smooth operation, the collected data must be annotated with information like the location of passersby, stop signs, traffic lights, and other vehicles.
Simply put: More annotated data = sharpened predictions = increasingly superior AI and machine learning models.
Here are some common types of data labeling and annotation to ensure predictive precision:
- Computer Vision: Assesses criteria in images and videos and compares them with similar new and unlabeled data, improving interpretations for predictive analysis
- Natural Language Processing (NLP): Evaluates written or spoken texts, captures meaning, determines patterns, and produces new text content
- Audio Processing: Converts and annotates all types of voices, from human speech to wildlife noises, for ML development
Best Practices for Data Labeling and Annotation
We now know that top-level AI and ML typically revolve around high-quality data, which can be achieved through data labeling and annotation. Nevertheless, maximizing the full potential of human-annotated data demands adherence to certain best practices to enhance the quality, accuracy, and efficiency of data labeling and annotation.
What are the best data labeling and annotation practices that propel you to the top of the dynamically uphill tech landscape?
➡️ How Do I Start With My Outsourcing? Book A Free Call
Intuitive and streamlined task interfaces
While data annotation makes data preparation seamless, getting there may be tedious, especially with a single database storing thousands of unlabeled data.
An intuitive interface can boost the rate of labeled data, enabling annotators to optimize the process, enhance annotation quality, and effectively organize annotation projects for ideal AI and ML models. From an image annotation vantage point, user-friendly features can reduce cognitive load on image labels, enabling quick and precise tagging. Supervised learning is a critical technique for this interface, which trains algorithms to classify data and predict outcomes.
Consensus
Even experts can disagree on a label, resulting in bias and inaccuracies that adversely affect your model’s performance. To resolve this conflict and reconcile the differing labels, there needs to be an agreement or convergence on the correct or most appropriate label for a specific piece of data.
As such, several annotators will review and cross-validate the same data independently and then attempt to arrive at a similar or identical label through a consensus (determined by metrics). Achieving this consensus mitigates errors, reduces subjectivity and ambiguity, and enhances the overall quality of annotated datasets.
One of the metrics used to measure consensus is by calculating the consensus score: dividing the total number of agreeing labels by the sum of labels per asset. The label obtaining the highest score will be considered ideal for data labeling and annotation.
Label auditing
Aside from gauging the degree of agreement among different sources, a consistent and systematic review of the label types is imperative to keep abreast of the quality and abide by specific standards or guidelines, leading to more accurate and robust machine learning models. It’s also a significant step in ascertaining the reliability of labeled datasets for training machine learning models or AI systems.
Transfer learning
Collecting and training massive data can be daunting, especially if you’ve just begun your AI and ML journey. Without prior experience or well-trained skills, you may set yourselves up for unnecessary failure, derailing all the other processes you’ve worked hard for.
Fortunately, you can avoid this mishap by adopting transfer learning.
Transfer learning is a machine learning technique where a pre-trained model is repurposed or adapted to perform other related tasks with a different but related dataset. It involves leveraging knowledge gained from solving one problem and applying it to a similar yet distinct problem domain.
A little goes a long way with this approach. Since the model has learned from a task with rich labeled data, a small amount of data is all you need to empower your models to perform at a high level.
Active learning
Data labeling and annotation services entail a time-consuming yet laborious process. Imagine spending time just manually inputting enormous datasets. Not only will this slow down your ML and AI progress, but you will also miss out on lucrative opportunities to expand your business ventures.
Fortunately, thanks to the magic of active learning, you can accelerate your data labeling and annotation process and reduce manual efforts.
Instead of marking random data, active learning streamlines learning by selecting the most informative or relevant data to label or annotate, maximizing learning efficiency and making the process more data-centric. Some of the active learning approaches include:
- Membership query synthesis: Produces an example of data for labeling
- Pool-based sampling: Ranks all unstructured data based on informativeness and relevance
- Stream-based selective sampling: Selects the unlabeled subset of data and decides which ones are the best fit for annotation
Outsourcing
The U.S. is still battling a persistent labor shortage, with 30.5 million professionals leaving their jobs as of August 2023. Naturally, the talent crunch will trickle down across all industries, including the data annotation and labeling fields.
So, how can companies tap into a qualified workforce amid labor scarcity? By outsourcing data annotators from a reliable and trustworthy outsourcing company.
Outsourcing data labeling and annotation can be a strategic move for businesses aiming to accelerate AI development, reduce costs, enhance accuracy, and focus on core competencies. Simultaneously, companies can request comprehensive information on their workers to facilitate the vetting process and choose ones who can deliver exceptional results.
How Outsourcing Data Labeling and Annotation Can Give You a Competitive Edge
The advancement of AI and ML has been extraordinary, transforming them into instrumental tools for long-term business growth. To cushion the impacts of labor scarcity and the economic downturn, many companies invest in outsourcing strategies to enjoy the “best of both worlds” — access to a team of well-trained data annotators at a cost-efficient rate.
Let’s take a closer look at the several benefits of outsourcing data annotation services:
➡️ Not Sure Whether You’re Ready To Outsource? Send Us an Email
Cost Efficiency
A 2020 research revealed that 70% of respondents partner with third-party vendors to minimize cost, making it the primary driver of outsourcing. Let’s compare the average annual salary in the U.S. and Colombia, a nearshoring powerhouse. The average yearly salary for data annotation specialists in the U.S. is $49,000, whereas in Colombia, it’s only $9,337.91 (COP 37,135,828).
In this example alone, companies can save up to five times more if they outsource from a competent BPO company, which can help alleviate the effects of the economic collapse.
Faster Turnaround Time
Say your phone is broken and requires urgent repair. The technician informs you to return in three days to claim it. You do as you’re told to discover that your phone is still being repaired.
Understandably, you feel frustrated because of the delay. The same applies to your clients.
This is why time is imperative. Beating project deadlines while maintaining quality is critical to securing viable annotations. Failure to do so can create a ripple effect, forestalling the other components of your AI and ML operations.
Outsourcing can be the oil that lubricates your business gears, enabling them to run smoothly and efficiently. Given the specialization, you can benefit from its streamlined workflow for quicker turnaround times and overcoming data labeling bottlenecks.
Focus on Core Business Activities
The responsibilities of data annotation and labeling specialists don’t just stop with inputting metatags to data. They work closely with the research and development team to discuss data annotation issues and come up with solutions.
With data annotation and labeling being a crucial piece of the puzzle in the ML lifecycle, it’s best to entrust this task to the capable hands of your outsourced experts.
Furthermore, outsourcing labeling tasks frees up in-house resources, allowing your team to focus on core tasks such as model development, research, and innovation. Consequently, businesses can adapt more quickly to fluctuating market demands and emerging trends.
Improved Data Protection and Quality
Since quality data is the linchpin of data annotation, businesses can’t afford to be precarious about their security and standard measures. With the cost of a data breach hitting an all-time high at $4.45 million, it’s more crucial than ever for companies to fortify their data protection.
For outsourcing partners, data protection has become non-negotiable. These professionals safeguard transparency and informed consent in data collection while utilizing anonymization methods to ensure utmost privacy. Additionally, they diligently analyze DMARC reports to strengthen email security, proactively addressing potential vulnerabilities and minimizing the risk of unauthorized access.
Furthermore, they implement comprehensive and straightforward rules to guide human annotators in ascertaining high-quality and consistent annotations. For instance, the guidelines provide examples of labeled data and standard data classifications to avoid irregularity in work ethics and practices.
Scalability and Flexibility
Hiring in-house data annotators can help maintain your employee pipeline. However, not all annotation tasks are meant for the long term. Companies may incur more losses if they’re not strategic about their recruitment plan.
Instead, businesses can outsource data annotators, easing the scaling up or down process based on their project demands and ensuring flexibility in managing varying data volumes. They gain access to a diverse pool of annotators with different skill sets and expertise for various data types and domains.
Outsourcing allows for easy scaling up or down based on project demands, ensuring flexibility in managing varying data volumes. It also provides access to a diverse pool of annotators with different skill sets and expertise for various data types and domains.
Advantages of Nearshoring Data Labeling and Annotation from Colombia
Colombia has become an epicenter of tech advancements and social innovation, making the country a hub for nearshoring roles indispensable for AI and ML development. One of which is data labeling and annotation.
Why should you outsource data labeling and annotation services in Colombia?
High Confidence Rate
The 2023 BPO Confidence Index reveals that Colombia remains one of the top countries gaining confidence among businesses, scoring 82.6%. Furthermore, the LatAm nation garners excellent ratings for the availability of front-line reps (90%), supervisors (87.5%), and operational leaders (82.5%).
Favorable BPO Legislations
As a strategy to attract direct foreign investments, Colombia offers tax incentives, Free Trade Zones, and other investment benefits. If you’re in the IT industry and investing in Colombia’s science, technology, and development projects, you may enjoy a 25% tax discount and a 100% tax deduction.
Skilled Workforce
While proficient in English, the labor pool also boasts exceptional technical skills due to its reformed education system. For instance, schools have integrated digital devices into their teaching to instill digital knowledge.
Geographical Proximity
Dubbed the “Gateway of South America,” the geographical advantage allows real-time collaboration and contributes to the profound understanding and cultural affinity with U.S. teams. A direct flight from Washington D.C. to Bogota, Colombia, only takes about six to seven hours, enabling in-person meetups anytime.
Unlock Precision: Partner with SuperStaff for Accurate Data Labeling and Annotation Services
➡️Let Us Know What You Need. Contact Us
Indeed, data has revolutionized AI and ML systems, from ensuring accurate predictions to guiding business decisions. Inevitably, leveraging data labeling and annotation capabilities stands at the forefront of high-quality data production and innovating top-notch intelligence models.
Allow SuperStaff to empower your AI and ML journey.
Our team can help you maximize your overall data labeling experience, bringing together a qualified workforce, industry-level skills, and superior quality to transcend challenges and enhance data training processes. Embark on your AI and ML journey with our SUPER team today and see how we can unlock your potential!